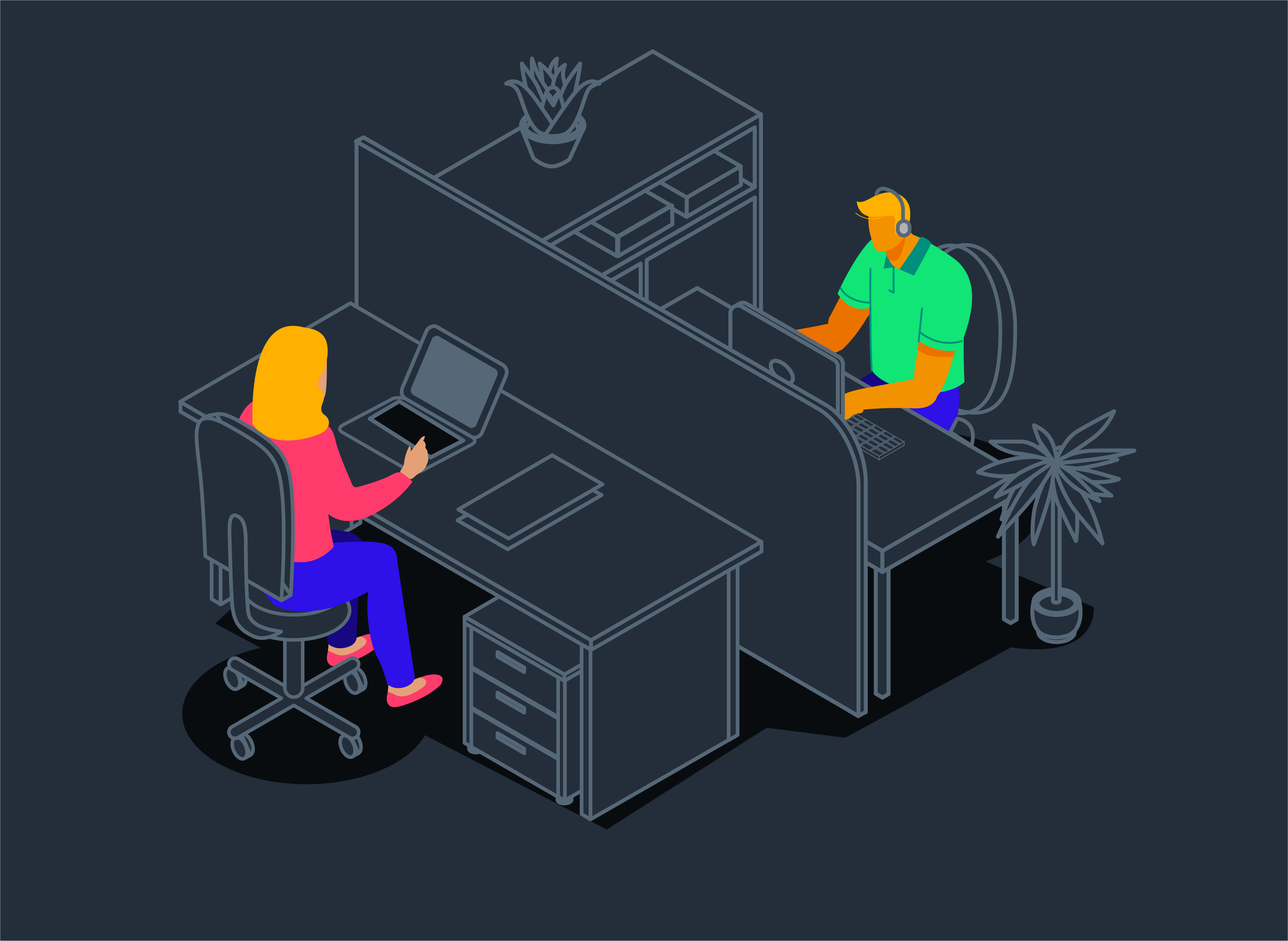
Credit risk is the possibility a lender will default on a loan extended by a bank or financial institution. The use of artificial intelligence (AI) in credit risk management enables banks to predict the probability of a counterparty defaulting by leveraging on both traditional and alternative data sources. The probability insights provide a basis for banks to decide whether to avoid extending a loan or extend the loan with mitigations such as higher interest rates or collateral.
Credit risk modeling is the means by which banks use data models to predict credit risks. The models when queried indicate the probability of a borrower defaulting and the corresponding impact of the default on the lender financials. Credit risk modeling has three main components: Probability of Default (POD), Loss Given Default (LGD) and Exposure at Default (EAD). POD quantifies the likelihood of default over a specific time horizon, usually one year. LGD on the other hand, is the loss a bank will incur when a borrower defaults, depicted as a percentage of the total loan portfolio (all the loans extended by the banks). EAD is the total loss exposure in terms of currency, of the lender at a point of loan default.
All the three credit parameters are measured using analytics, which often suffer from data quality challenges. The Targeted Review of Internal Models (TRIM) 2021 report, indicated that 71% of banking institutions investigated suffer from a poor data quality system, procedures and processes. The shortcomes identified range from data quality monitoring and mechanisms in place useful to identify, remedy and follow-up on data quality issues. Since the data is used for POD and LGD modelling, that number is quite significant. Diversity of data sources and availability of corporate wide data compound the challenge of quality.
But AI is changing credit risk management. Availability of unstructured data and the improvement in computing power make machine learning, a subset of artificial intelligence, a viable means of credit risk models. Legacy IT infrastructure systems in banks use rule-based artificial intelligence systems that are cumbersome, requiring substantial manual effort to clean and analyse the data. The systems draw data from selected sources which provide a partial view of the customers credit history without contextualising within the framework of the wider market activities.
However, with machine learning systems, banks will be able to process copious amounts of structured and unstructured data from multiple sources and surface trends, and deviations from expected behaviour. Machine learning systems implement rules that learn and adapt to changes in the environment. Instead of looking through a narrow lens of historical data collected internally, banks can now benefit from a comprehensive, continuous flow of insights based on both historical and alternative data.
While legacy systems depend on traditional data sources such as company financials, with ML systems using alternative data, banks can detect risk factors early as opposed to navigating the landscape when risk becomes reality.
AI in credit risk management is also instrumental in solving data quality challenges. AI systems can be deployed to highlight certain outliers in the data and offer remedial recommendations. Also due to the complexity and the centrality of data in credit modeling, banks have opted to set up a data department to support the credit risk modeling process. Big data analytics help banks make a better predictor of POD than rule-based legacy systems. Artificial intelligence and machine learning are also useful for helping banks validate their modelling systems.
The centrality of data in credit modeling means sound data governance systems determine the accuracy of the process. Credit risk models are used to measure a bank’s capital requirement, impairment provisions and forecasting and stress testing.
Tools such as AMPLYFI’s DeepInsight, which leverages on unstructured data, can be useful in complementing existing credit risk models. For more information, book a free, no-obligation consultation with one of our experts.